=Overview
We study the inverse graphics problem of inferring a holistic representation for natural images. Given an input image, our goal is to induce a neuro-symbolic, program-like representation that jointly models camera poses, object locations, and global scene structures. Such high-level, holistic scene representations further facilitate low-level image manipulation tasks such as inpainting. We formulate this problem as jointly finding the camera pose and scene structure that best describe the input image. The benefits of such joint inference are two-fold: scene regularity serves as a new cue for perspective correction, and in turn, correct perspective correction leads to a simplified scene structure, similar to how the correct shape leads to the most regular texture in shape from texture. Our proposed framework, Perspective Plane Program Induction (P3I), combines search-based and gradient-based algorithms to efficiently solve the problem. P3I outperforms a set of baselines on a collection of Internet images, across tasks including camera pose estimation, global structure inference, and down-stream image manipulation tasks.
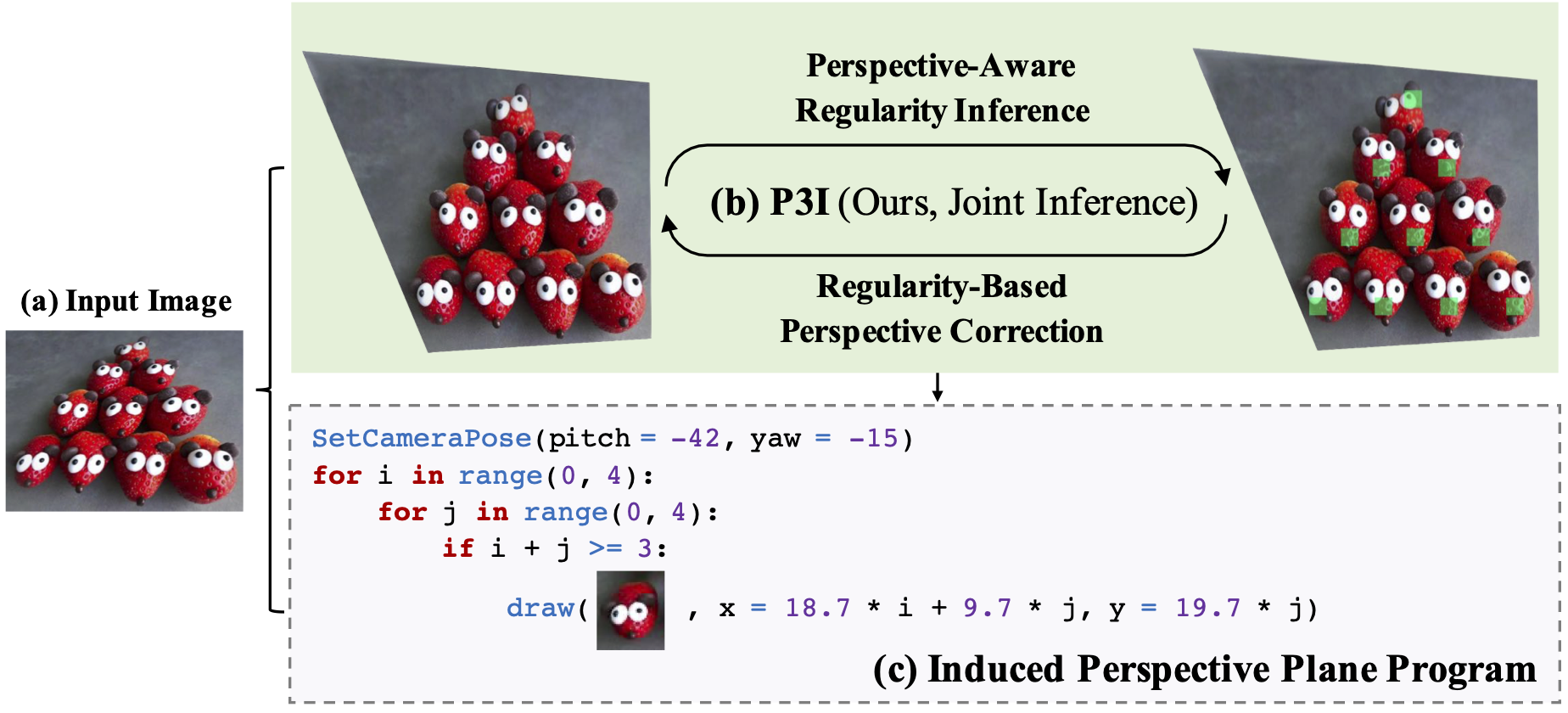
Figure 1: Perspective effects and scene structure regularity are ubiquitous in natural images (a). We propose to jointly solve perspective correction and regularity structure detection (b) by simultaneously seeking the program and perspective parameters that best describe the image (c).
=Resources
- Code in [Python].